Earth Species Project, a non-profit organization dedicated to decoding animal communication, is at the forefront of this groundbreaking research. By leveraging machine learning, advanced signal processing, and artificial intelligence, they aim to develop a comprehensive understanding of non-human communication and foster empathy between humans and other species. Their ambitious goal is to create open-source AI tools to translate animal languages, paving the way for meaningful interspecies dialogue.
We are currently engaged in a number of critical AI research projects which feature on this roadmap and which have been developed in close partnership with our partners in biology and machine learning.
Technology – Creating unified benchmarks and data, acoustic and multimodal, to validate our experiments and accelerate the field, vetted by top biologists and AI researchers.
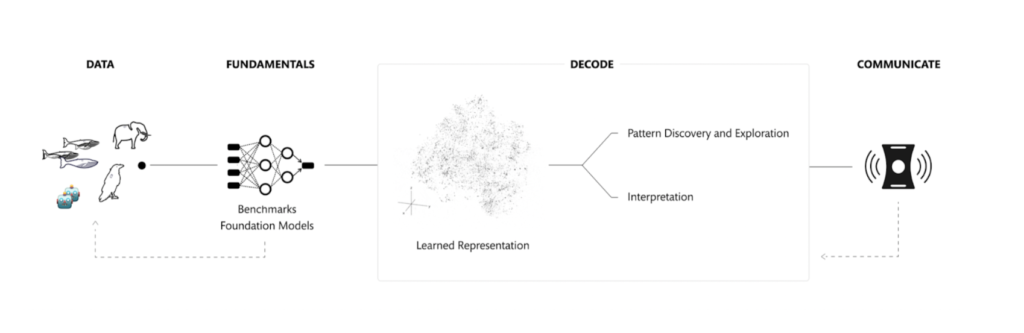
Turning motion into meaning — automatic behavior discovery from large-scale animal-borne tag data
Training self-supervised foundation models such as HuBERT and evaluating them against our benchmarks
Establishing semantic generation and editing of communication — which will ultimately allow for the creation of new signals that carry meaning and unlock two-way communication with another species.
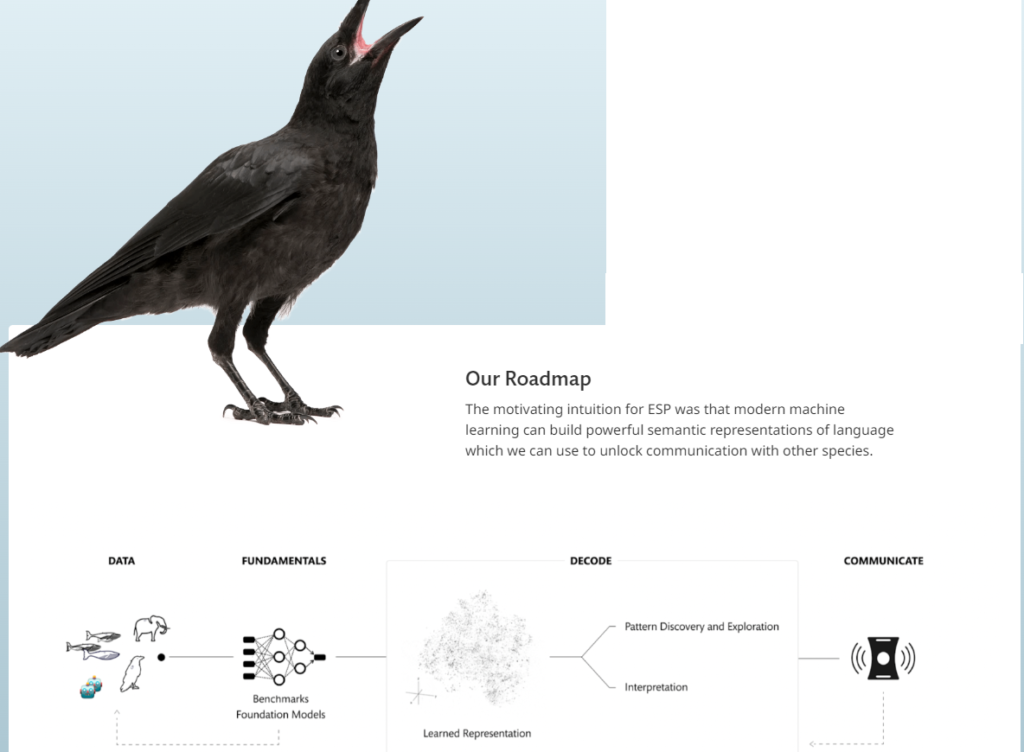
Understanding and decoding animal communication is a complex and fascinating field of study. Animals communicate with each other using a variety of signals and cues, including vocalizations, body language, chemical signals, and even electrical signals. Deciphering these forms of communication can provide insights into the behavior, social structure, and ecology of different species. Here are some key points related to this field:
- Vocalizations: Many animals use vocalizations to communicate with each other. For example, birds sing to establish territory or attract mates, and dolphins use clicks and whistles for social interactions. Researchers use spectrograms and audio analysis software to study these vocalizations and their meaning.
- Body Language: Animals also communicate through body language, including posture, gestures, and facial expressions. Observing and interpreting these cues can help researchers understand social hierarchies, mating behaviors, and more.
- Chemical Signals: Some animals release chemical signals, known as pheromones, to convey information to other members of their species. These signals can be used for marking territory, attracting mates, or signaling danger.
- Electrical Signals: Certain animals, such as electric fish, use electrical signals to communicate and navigate their environments. Researchers study the electrical fields generated by these animals to decode their communication methods.
- Technology and Research Tools: Decoding animal communication often involves advanced technology, including specialized recording equipment, video analysis software, and genetic analysis to understand the underlying biology of communication.
- Behavioral Studies: Ethologists and behavioral ecologists conduct field research and controlled experiments to decode animal communication. This involves observing animals in their natural habitats or controlled environments.
- Conservation and Wildlife Management: Understanding animal communication can have practical applications in wildlife conservation and management. For example, it can help researchers develop strategies to protect endangered species, manage wildlife populations, and reduce human-wildlife conflicts.
- Cross-Species Comparisons: Comparing communication across different species can provide insights into the evolution of communication systems and shed light on the commonalities and differences between human and animal communication.
Projects:
Generative Vocalization Experiment
Playbacks are a common technique used to study animal vocalizations, involving the experimental presentation of stimuli to animals (usually recorded calls played back to them) to build an understanding of their physiological and cognitive abilities. With current playback tools, biologists are limited in their ability to manipulate the vocalizations in ways that will establish or change their meaning, and their exploratory power is limited. Senior AI research scientist Jen-Yu Liu is exploring whether it is possible to train AI models to generate new vocalizations in a way that allows us to solve for a particular research question or task.
Jen-yu has been working on generating calls for a number of species, including chiff-chaffs (Phylloscopus collybita) and humpback whales (Megaptera novaeangliae). A new project is now allowing us to test this work through interactive playback experiments with captive zebra finches (Taeniopygia guttata) in partnership with research scientist Dr. Logan James at the University of McGill. Providing researchers with the ability to control various aspects of the vocalization production process will greatly expand the exploratory and explanatory power of bioacoustics research, and is an important step on our Roadmap to Decode.Given that we may not understand the meaning of the novel vocalizations being generated by the model, there are important ethical considerations related to potentially interfering with animals and their culture. For this reason, we are beginning this research only with captive populations and working exclusively with scientists who follow strict ethical protocols.
Crow Vocal Repertoire
Senior AI Research Scientists Benjamin Hoffman, Maddie Cusimano and Jen-Yu Liu are working to map the vocal repertoires of two species of crow. The first one, the Hawaiian crow, is notable for its natural ability to use foraging tools as well as its precarious conservation status – the species sadly became extinct in the wild in 2002 and currently only survives in captivity. With Professor Christian Rutz and collaborators we are investigating how the species’ vocal repertoire has changed over time in two captive breeding populations, to inform ongoing reintroduction efforts. The second species, the carrion crow, is abundant across its European range, but has attracted attention with its unusually plastic social behavior, with groups in some populations breeding cooperatively. We are working with Professors Daniela Canestrari, Vittorio Baglione and Christian Rutz to analyze field recordings to understand the role of acoustic communication in group coordination. Mapping vocal repertoires can help uncover cultural and behavioral complexity, which in some cases has important implications for planning effective conservation strategies.
Vocal Signaling in Endangered Beluga Whales
Sara Keen, Senior Research Scientist, AI and Behavioral Ecology is working with Jaclyn Aubin at the University of Windsor to explore whether we can use machine learning to categorize unlabeled calls of an endangered population of beluga whales (fewer than 1,000 remaining) in the St. Lawrence River, Canada, in order to help determine vocalization repertoire size. She hopes to eventually quantify the similarity of contact calls recorded at different sites, with the goal of determining whether dialect patterns are present. The ultimate goal of the project is to drive a better understanding of the social structure of the whales in order to help minimize human impacts on them.
Self-Supervised Ethogram Discovery
Senior AI research scientist Benjamin Hoffman, supported by Maddie Cusimano is working on self-supervised methods for interpreting data collected from animal-borne tags, known as bio-loggers. Using bio-loggers, scientists are able to record an animal’s motions, as well as audio and video footage from the animal’s perspective. However, these data are often difficult to interpret, and there is often too much data to analyze by hand. A solution is to use self-supervised learning to discover repeated behavioral patterns in these data. This will allow behavioral ecologists to rapidly analyze recorded data, and measure how an individual’s behavior is affected by external factors, such as human disturbance or communication signals from other individuals. Ben is working closely with our partners, Dr. Christian Rutz and Dr. Ari Friedlaender, to source datasets from their labs and among researchers in the ethology community.
Benchmark of Animal Sounds (BEANS)
Machine learning is increasingly being used to process and successfully analyze bioacoustic data in ways that support research projects. However ongoing challenges include the diversity of species being studied, and the small data sets compared to human language data sets. Machine learning approaches have also been very specific, making it difficult to compare across species and models. Senior AI Research Scientist Masato Hagiwara has developed a benchmark for bioacoustics tasks that measures how a model can perform over a diverse set of species for which there is comparatively little data. This mirrors the standard benchmarks which have been developed for human vision and language. The development and publication of this benchmark is supporting the fair comparison and development of ML algorithms and models that do well for a diverse set of species with small data; lowers barriers to entry by open-sourcing preprocessed datasets in a common format, infrastructure code, and baseline implementations; and encourage data and model sharing by providing a common platform for researchers in the biology and the machine learning communities. This benchmark and the code is open source in the hope of establishing a new standard dataset for ML-based bioacoustic research.