Embryonics is developing and applying data-driven solutions to improve the journey and the success rates of fertility treatments.
The process of fertilizing a human egg outside the body in order to help those suffering from infertility to conceive is known as in vitro fertilization (IVF). Despite being the most effective method of assisted reproductive technology (ART), the average success rate of IVF is a mere 20-40%. One step that is critical to the success of the procedure is selecting which embryo to transfer to the patient, a process typically conducted manually and without any universally accepted and standardized criteria.
In vitro fertilization (IVF) is a procedure in which ova (egg cells) harvested from an adult female are fertilized by live sperm in vitro. After successful fertilization, the resulting embryos are incubated for several days while a trained embryologist manually tracks their development, using morphological and/or morphokinetic characteristics to generate a grade
for each embryo indicative of its viability and likelihood of successful uterine implantation and, hopefully, live birth.
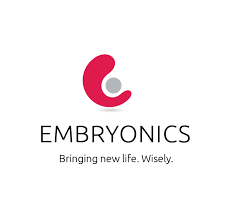
Although manual morphological annotation and quality assessment of embryos fertilized in vitro remains the gold standard for predicting IVF success, efforts to standardize and improve prediction accuracy have become increasingly computational.
Most algorithms developed for embryo outcome prediction require user-defined input parameters (such as specific morphological characteristics), execute a series of user-defined tasks, and then produce an estimated probability of achieving a user-defined outcome. Essentially, this approach can be seen as an attempt to mimic the human embryologist. While algorithms of this nature may help embryologists to more efficiently assess embryo quality, they are limited in their ability to improve outcomes as they are often dependent on the same scoring parameters as manual assessment, which is highly variable between observers.
Team used novel data-driven system trained to directly predict embryo implantation probability from embryogenesis time-lapse imaging videos. Using retrospectively collected videos from 272 embryos, to demonstrate that, when compared to an external panel of embryologists, their algorithm results in a 12% increase of positive predictive value and a 29% increase of negative predictive value.