Atmospheric radiative transfer models simulate the radiative transfer interactions of light scattering and absorption through the atmosphere. These models are typically used for the atmospheric correction of airborne/satellite data and allow retrieving atmospheric composition.
Numerical weather prediction (NWP) are poised to take advantage of recent years’ rapid advances in ML.
To adapt ML to weather-related applications, it is critical to meet all of these needs at multiple spatial and temporal scales for diverse geophysical domains that include the atmosphere, ocean, biosphere, hydrosphere, and near-space environment.
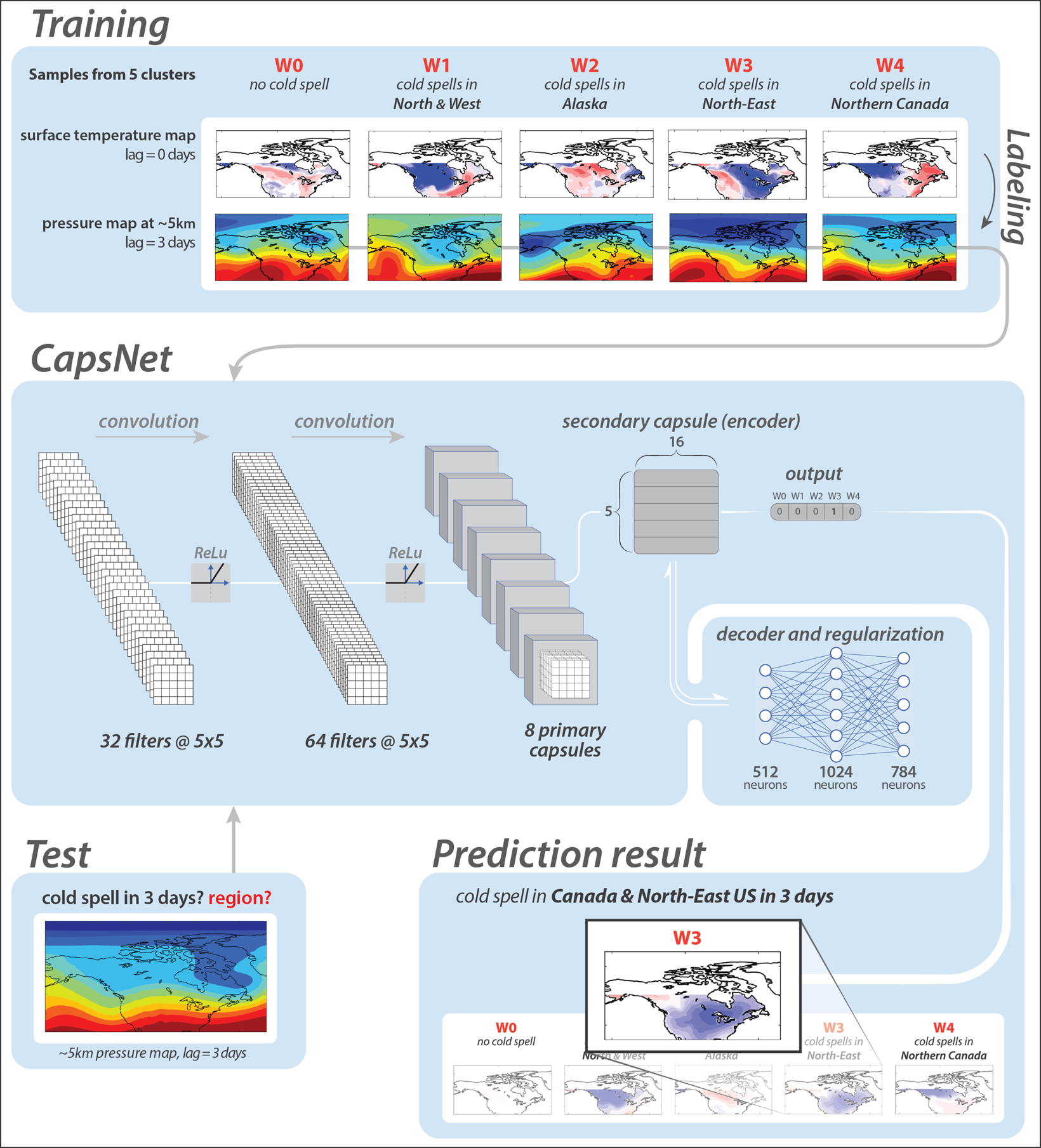
Typically, the heating and cooling of the atmosphere are calculated for one particular time and then held fixed, in some cases for hours. Meanwhile, the other atmospheric processes are simulated with time steps of a few minutes.
At the core of a radiative transfer model lies the radiative transfer equation that is numerically solved using a solver such as a discrete ordinate method or a Monte Carlo method.
The radiative transfer equation is a monochromatic equation to calculate radiance in a single layer of the Earth’s atmosphere. To calculate the radiance for a spectral region with a finite width (e.g., to estimate the Earth’s energy budget or simulate an instrument response), one has to integrate this over a band of frequencies (or wavelengths). The most exact way to do this is to loop through the frequencies of interest, and for each frequency, calculate the radiance at this frequency.
ML is capable of extracting information from large data sets and establishing and approximating complex relationships between disparate data sets of different types (physical, chemical, and biological, to name a few).
For this, one needs to calculate the contribution of each spectral line for all molecules in the atmospheric layer; this is called a line-by-line calculation. For an instrument response, this is then convolved with the spectral response of the instrument. A faster but more approximate method is a band transmission. Here, the transmission in a region in a band is characterized by a set of pre-calculated coefficients (depending on temperature and other parameters).
A fast radiative‐transfer model is tested at ECMWF: NeuroFlux. It is based on an artificial neural‐network technique used in conjunction with a classical cloud approximation (the multilayer grey‐body model). The accuracy of (he method is assessed through code‐by‐code comparisons, climate simulations and ten‐day forecasts with the ECMWF model. The accuracy of NeuroFlux appears to be comparable to the accuracy of the ECMWF operational scheme, with a negligible impact on the simulations, while its computing time is seven times faster.
Credit :
Homepage
RMetS
Nasa.gov